Enhanced Data Governance For Better Customer Remediation
In our last article we looked at some of the common challenges and opportunities Australian Financial Services Licensees (AFSL’s) have to improve efficiency when remediating customers.
In this article we take a more detailed look at how AFSL’s can enhance data governance in the context of customer remediation.
Dealing with poorly managed historical data, extracting data from legacy systems or archives is a common challenge for AFSL’s, particularly when undertaking customer remediation. Below are some tips to handle these situations.
Tip 1: Data Mapping and Cataloguing
These processes, that are best applied strategically, but also pay dividends tactically inside a customer remediation project, lay the groundwork for effective data governance and use. Here’s a step-by-step guide:
Cataloguing Data Assets
Develop a comprehensive catalogue of data assets, documenting their structure and content.
Step 1: Inventory Existing Data Systems
Begin with an inventory of all data repositories, legacy and current. This includes product systems, databases, data lakes, file systems, cloud storage solutions, and any other platforms where data may reside.
Step 2: Document Data Assets
For each identified system, document the types of data stored, including the format and content. This could range from customer information to transaction or compliance records.
Step 3: Assess Data Quality
Evaluate the quality of the data assets. Record any issues with accuracy, completeness, and timeliness that could impact remediation efforts.
Step 4: Data Asset Classification
Classify data based on its sensitivity, regulatory requirements, and criticality to business operations. This helps in prioritising data governance efforts.
Understanding Dependencies
Identify and document dependencies between legacy systems and the current operational systems.
Step 1: Identify Interdependencies
Determine how systems interact with each other. Look for data that is shared between systems and document the flow of data across these platforms.
Step 2: Visualise Data Flows
Create visual representations of data flows using data mapping tools. These diagrams should show how data moves from legacy systems to modern platforms and vice versa.
Step 3: Determine Impact
Assess how changes in one system could impact others. This step is critical for understanding the risks involved in data migration or system upgrades.
Step 4: Create a Dependency Matrix
Develop a matrix that outlines dependencies and indicates the level of impact an alteration in one system might have on another.
Tip 2: Data Aggregation
In the context of customer remediation, where AFSL’s must navigate through active, unconnected legacy, and even decommissioned systems to gather data, applying appropriate data processes is critical. Here’s a step-by-step guide:
Data Aggregation and Integration Layer
Develop an integration layer that aggregates data from various sources, including active systems, legacy databases, and archives from decommissioned systems.
Step 1: Implement an Integration Platform as a Service (iPaaS)
- Select an iPaaS solution that offers connectors for various data sources and types, ensuring compatibility with both current and legacy systems.
- iPaaS solutions can facilitate the rapid integration of data from multiple sources without the need for extensive coding.
Step 2: Create a Unified Data Model
- Design a unified data model that represents all the data elements needed for customer remediation across different systems.
- Use this model to map data fields from each system into a standardised format that can be used for analysis and reporting.
Step 3: Automate Data Extraction
- Develop automated scripts or use ETL tools to extract the necessary data from each system based on the unified data model.
- Schedule regular extractions to keep the aggregated dataset up-to-date.
Data Quality Enhancement
For remediation, the quality of data is paramount as it directly impacts the ability to identify affected customers and calculate compensation.
Step 1: Data Cleansing
- Deploy data cleansing tools that can detect and correct errors in the data, such as inconsistencies or duplicate records.
- Perform data profiling to understand the quality of data and identify areas that require cleansing.
Step 2: Data Enrichment
- If customer data is incomplete, use data enrichment services to fill in the gaps, such as updating contact details to facilitate customer communication.
- Verify the enriched data against multiple sources to ensure accuracy.
Step 3: Data Validation
- Implement validation rules based on the remediation criteria to ensure all necessary data for customer identification and compensation calculation is present and accurate.
- Use validation reports to prioritise data quality efforts on the most impactful issues.
Legacy System Data Utilisation
Dealing with data from legacy and decommissioned systems requires special attention due to their varied formats and potential quality issues.
Step 1: Data Archiving Solutions
- Use data archiving solutions to retrieve and store data from decommissioned systems in an accessible and secure environment.
- Ensure that the archiving solution complies with regulatory requirements for data retention and privacy.
Step 2: Legacy Data Wrangling
- Apply data wrangling tools specifically designed to handle the peculiarities of legacy system formats, converting them into a usable form for modern analysis.
- This step often involves manual intervention to decipher legacy data structures and encode them accurately into the current system’s understanding.
Step 3: Historical Data Analysis
- Perform historical data analysis to identify patterns and customer profiles that might have been impacted by past practices requiring remediation.
- This can uncover subsets of customers who were affected but might not be captured by current system data alone.
Tip 3: Packaging Data for Governance
Compiling and organising the data and associated documentation ensures that a customer remediation project is well-governed and stands up to internal and external scrutiny.
Compile a Comprehensive Data Package
The compilation of a data package is a critical activity, ensuring that all aspects of the remediation project are transparent and accountable. The package serves as a record of the work done, the decisions made, and the rationale behind those decisions. This is especially crucial for any third-party review or audit. Here’s a step-by-step guide:
Step 1: Identify Key Data Components
Determine all types of data that need to be included: transactional data, customer communication records, data analysis scripts, and quality assurance reports.
Step 2: Data Extraction
Extract the raw data and ensure that it includes metadata which provides context such as timestamps, source system identifiers, and data extraction methods.
Step 3: Document Analysis Steps
Clearly outline the data analysis process. Include query scripts, transformation logic, calculation methodologies, and any tools or software used.
Step 4: Quality Assurance Documentation
Add details of the quality assurance process, including the validation checks performed, issues identified, how they were resolved, and the final validation results.
Organise the Documentation
Organising the documentation methodically is crucial for good governance. It should present a coherent narrative of the project, allowing reviewers to follow the process from start to finish.
Good documentation practices not only facilitate audits and regulatory reviews but also contribute to institutional knowledge and can aid in training and process improvement initiatives.
Step 1: Develop a Structured Index
Create an index or table of contents that guides the reviewer through the package. Each section should be numbered and titled appropriately.
Step 2: Logical Sequencing
Arrange the documents in the order in which the activities occurred. This chronological sequencing helps in understanding the flow of the project.
Step 3: Cross-Referencing
Use clear references and hyperlinks between documents where actions in one document relate to or impact another.
Step 4: Summary Reports
Provide executive summaries for each major section, highlighting key actions, findings, and outcomes.
Step 5: Version Control
Ensure all documents are properly versioned with date stamps, and the final versions are included in the package.
Wrap-up
Strong data governance not only enhances the integrity and reliability of customer remediation projects but also supports regulatory compliance and better customer outcomes. It ensures that data utilised in the remediation process is accurate, and complete, directly influencing its fairness and effectiveness.
If you’d like to discuss any of these opportunities now, or explore support from CRCG, please reach out (contact us at info@crcg.com.au or click here to submit a request online).
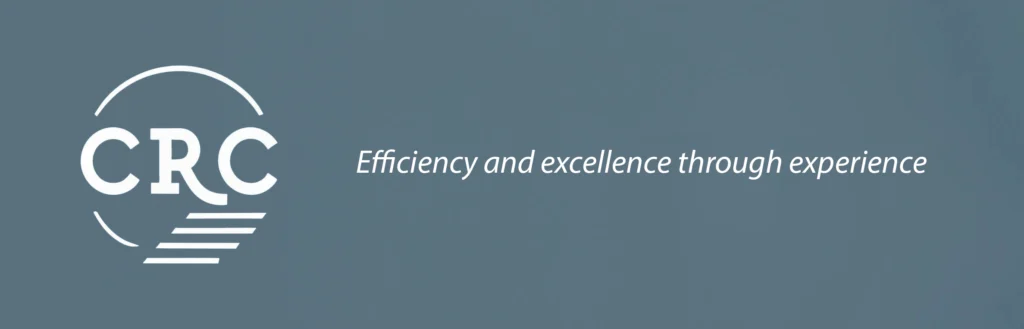
No responses yet